ChatGPT has been hot for a long time, and we have done some research to understand its investment opportunities. We share our findings with you here for discussion. Please note that our understanding may be limited by our expertise and we cannot guarantee complete accuracy. This article is written by BEDROCK member Jimmy and based on his team's discussion.
1、Large model evolution path
ChatGPT originated from the Transformer model, and we don't need to know too much about the details of the model. What's more important is to understand the source and speed of its ability improvement. It can be said that there are two modes of progress for large models: gradual and emergent.
Gradual progress: In a paper in 2020, OpenAI pointed out that before reaching the limit, with the exponential increase of model parameters, data volume, and computing power, although the ability of the model also increases exponentially, the speed of improvement is slow, as shown in the figure below:
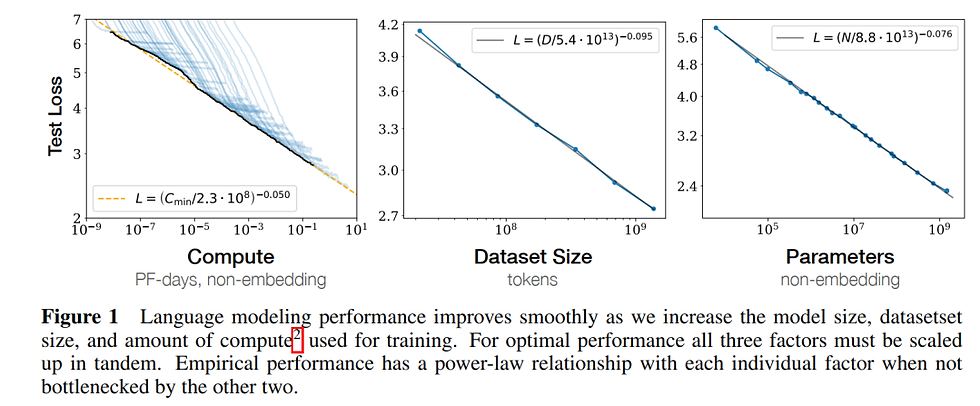
The horizontal axis represents computational power, data volume, and parameter size, while the vertical axis represents model performance (the smaller the better).
Emergence: In 2022, a paper from Google found that when the computational power of a model reaches a certain level, its processing ability for certain tasks can have an explosive improvement, as shown in the following figure:
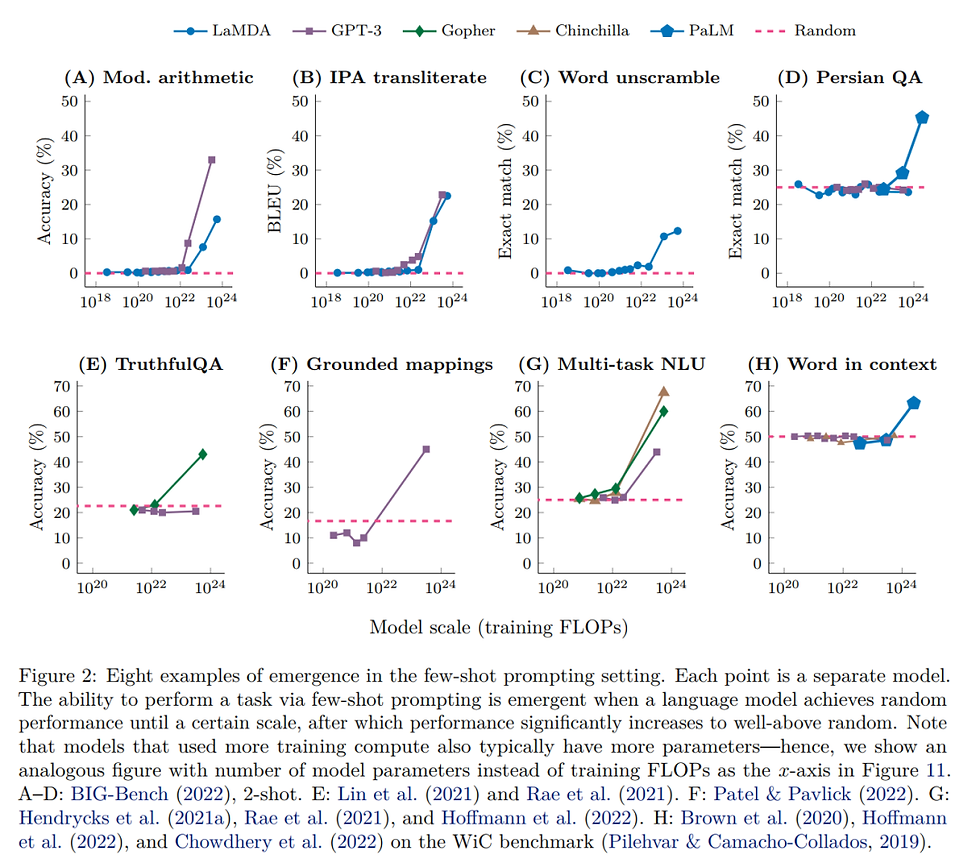
In other words, when the computing power of a large model reaches a certain level, it will suddenly learn some abilities, and this process is called emergence. Researchers have summarized various abilities that different large models have emerged, including addition and subtraction, chain-of-thought, and so on. It can be seen that the more complex the ability, the more computing power is needed for emergence (of course, it also depends on the training method).
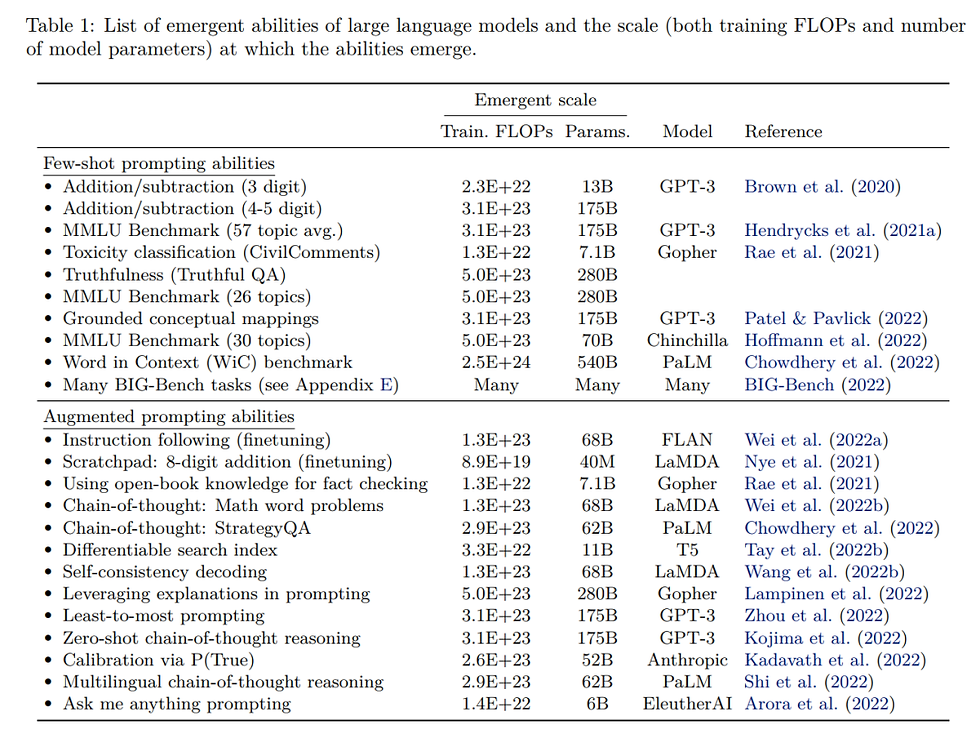
Among them, the chain-of-thought capability is shown in the following figure:
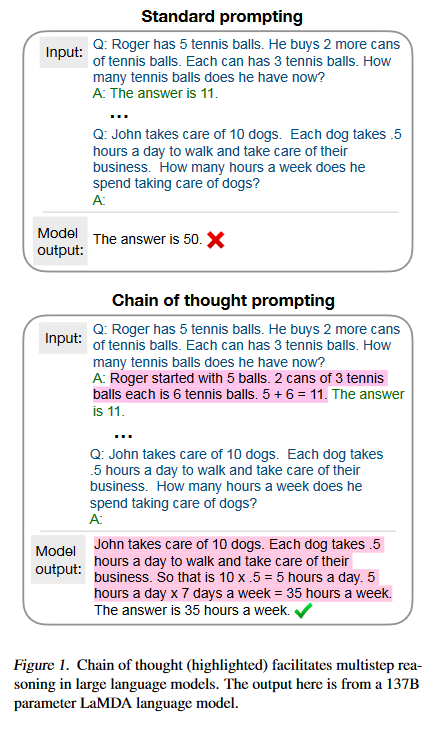
This leads us to the first conclusion: the computing power, parameter size, and data volume of large models will continue to increase, and we have not yet seen their peak.
2、The relationship between computing power, parameter volume and data volume
As the number of parameters and amount of data increase, the required amount of computing power undoubtedly increases as well. In the past, both the number of parameters and computing power have experienced a very rapid exponential increase.:
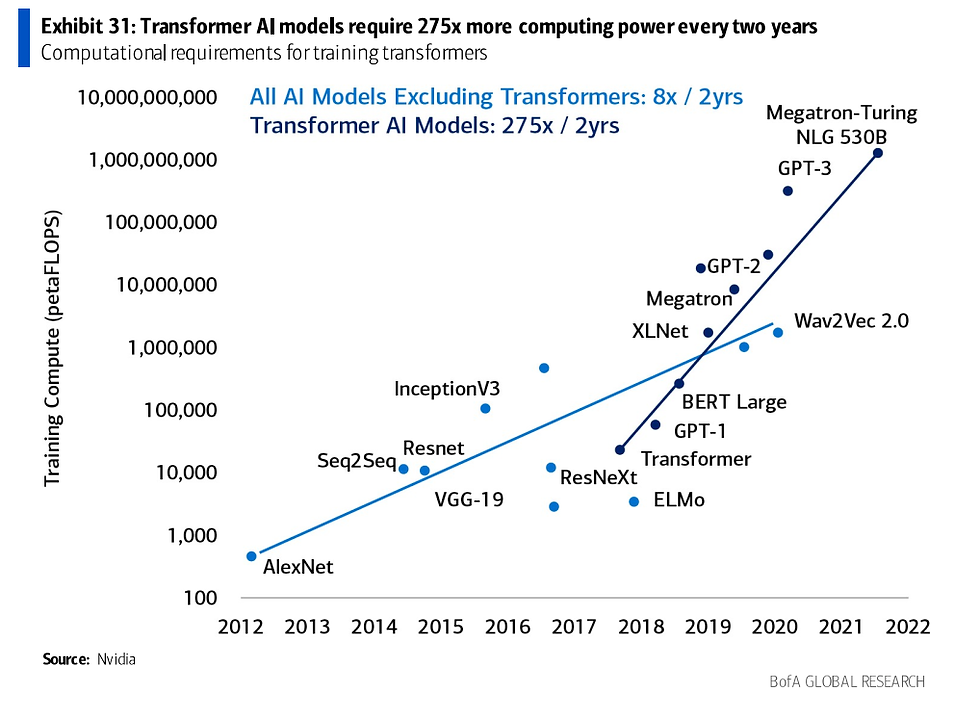
Exponential increase in computing power
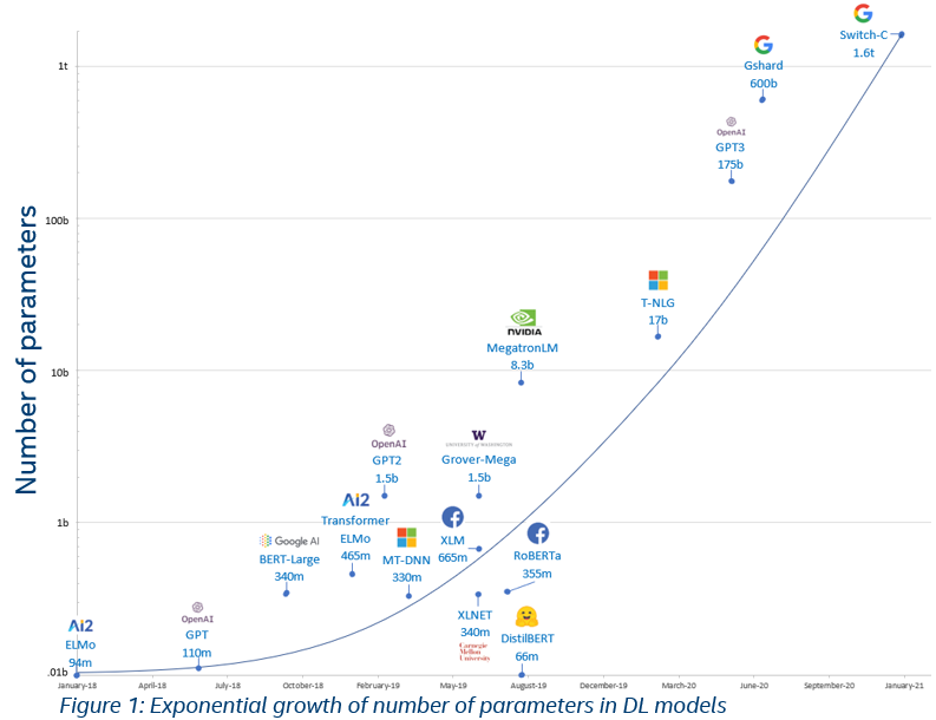
Exponential increase in the number of parameters
However, a 2022 paper from DeepMind found that when the computing power is fixed, the model parameter size is not always better, but has an optimal solution. Each point on each line on the left of the figure represents the same computing power, and it can be seen that when the number of parameters reaches a certain quantity, the performance of the large model reaches its maximum (the lowest point in the figure).
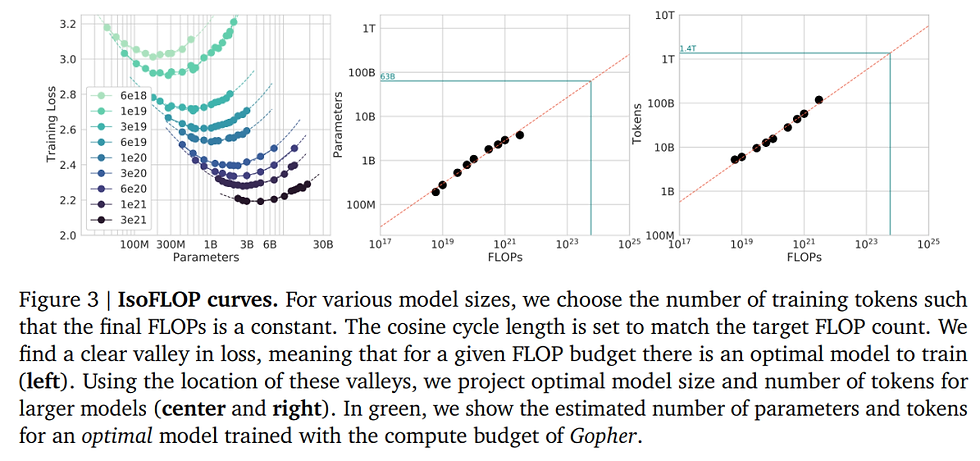
Based on this, we can draw the second conclusion: the performance of a large model depends on how much computing power is used during training, and adjusting the parameter count can only reveal the highest potential under the current level of computing power.
Therefore, when evaluating a large model, computing power rather than parameter count is a better indicator.
Additionally, according to the comparison results in the paper, it can be seen that GPT-3 has not yet achieved its optimal performance at its current parameter count. Therefore, we speculate that the parameter count of GPT-4 will be larger than that of GPT-3, but it will not have a 100x improvement like the jump from GPT-2 to GPT-3. Instead, it will increase the amount of training data to improve overall computing power usage and achieve an optimal state.

3、Investment Opportunities
AI industry TAM
The TAM (Total Addressable Market) of the AI industry is undoubtedly huge, but there are different ways of calculating it. We roughly calculated it based on the degree of improvement in human work efficiency brought about by AI:
By 2022, there will be 160 million employed people in the US, with an average annual salary of $50,000. Assuming that 30% of these people can improve their efficiency by 10% with the help of ChatGPT (only considering the current ChatGPT technology), and these people earn an annual salary of $80,000, then the value of the improvement would be $384 billion. Even if AI charges only 10% of this value, the TAM in the US would be $38 billion, and globally it would be around $80 billion.
If GPT continues to improve and can help 60% of people improve their efficiency by 30% in 25-30 years, with an average annual salary of $70,000, and AI charges remain at 10%, then the TAM in the US would be $200 billion, and globally it would be $400 billion.
Of course, this is only the value brought about by the improvement in efficiency in the existing industry with the help of AI. The value created by new industries and new functions created by AI cannot be estimated.
MSFT:
short term:
By the end of 2021, there will be 236 million Office subscribers (with 1.4 billion monthly active Windows users). Assuming 50% penetration of Office users and an annual subscription fee of $136, integrating GPT into various office software could increase subscription fees by $100 per year (the current price of ChatGPT Pro is $240), generating $12 billion in revenue, which is equivalent to 6% of Microsoft's revenue in 2022 ($198 billion).
In addition, Microsoft has said that 1% search engine market share corresponds to $2 billion in advertising revenue. If Microsoft can capture 10% of the search market (currently at around 3%), it could generate an additional $20 billion in revenue, equivalent to 10% of Microsoft's revenue in 2022 ($198 billion).
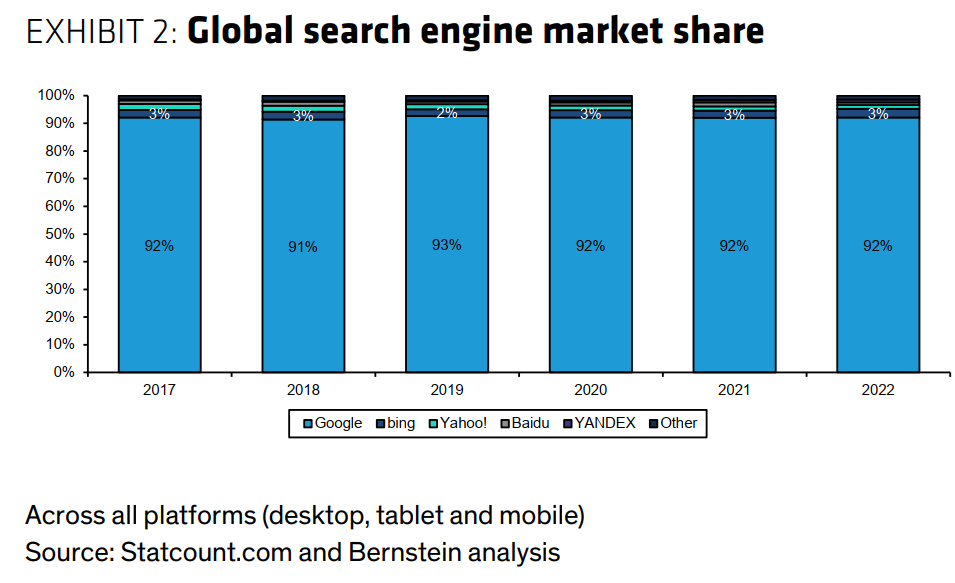
Long term:
In the office assistant market, assuming the number of Office AI users increases to 1 billion in 25-30 years (with 1.4 billion Windows users and a total of 1.7-1.8 billion including Mac users), with an annual AI subscription fee of $200, this would generate $2 trillion in revenue.
In the search market, assuming Bing's market share increases by 30% (reaching 33%), this would correspond to $600 billion in revenue.
For incremental markets such as AI chat and data monetization, there may also be $2 trillion in revenue.
Training
The demand for computing power by large AI models mainly comes from two areas: training and inference. Training corresponds to research and development costs, while inference corresponds to operational costs.
Specifically, in 2019, DeepMind lost about £500 million, and in 2022, OpenAI also lost $500 million. Therefore, the annual computing cost should be in the range of $300-400 million. Considering that there are not many companies capable and ambitious enough to develop large basic models, we assume that there are four OpenAI-level companies in 2022 and six in 2023 and beyond. The computing power demand of each company grows at a rate of 200%, while the demand for computing power decreases at a rate of about 40%. Based on this, we can calculate the capital cost required for AI training, and then convert it to the demand for hardware based on the business models of cloud computing companies. We can then calculate the impact on companies such as NVDA and TSM.
According to the calculation results, the contribution of training to NVDA's revenue is only 2-3%, and less than 1% for TSM. Therefore, it can be said that the impact is not decisive.
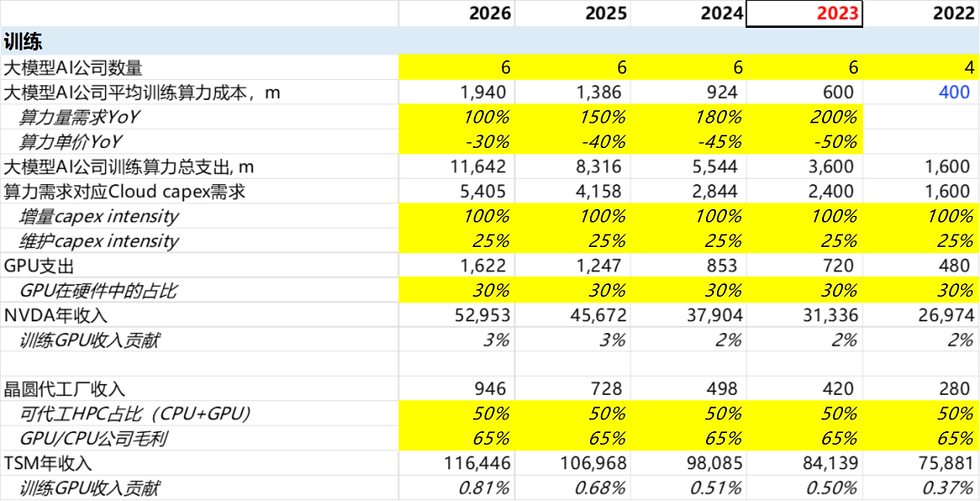
Inference
When large AI models are applied on a large scale, the cost of inference will increase significantly, and it will increase rapidly as AI is promoted. We have made rough estimates of the opportunities brought about by inference in AI applications such as search, chat, and office.
In our estimation, we assume that the penetration rate of Microsoft's new Bing search will increase to 20% by 2026, and that the cost of AI inference will decrease rapidly in 2023 (as it is the first commercial application and there is a lot of room for cost reduction). Although OpenAI has announced a 10x reduction in the pricing of ChatGPT, we do not believe that this is a realized result but rather a low-price strategy to quickly capture the market.
We expect that in the next few years, AI inference will contribute to 23% of Microsoft's revenue, 7% to NVDA's revenue, and only 3% to TSM's revenue. This means that downstream applications (such as Microsoft) benefit the most from OpenAI, followed by hardware such as GPUs (such as NVDA), and finally semiconductor fabs such as TSM, which is in line with the characteristics of the semiconductor industry, with few upstream players and more downstream players.
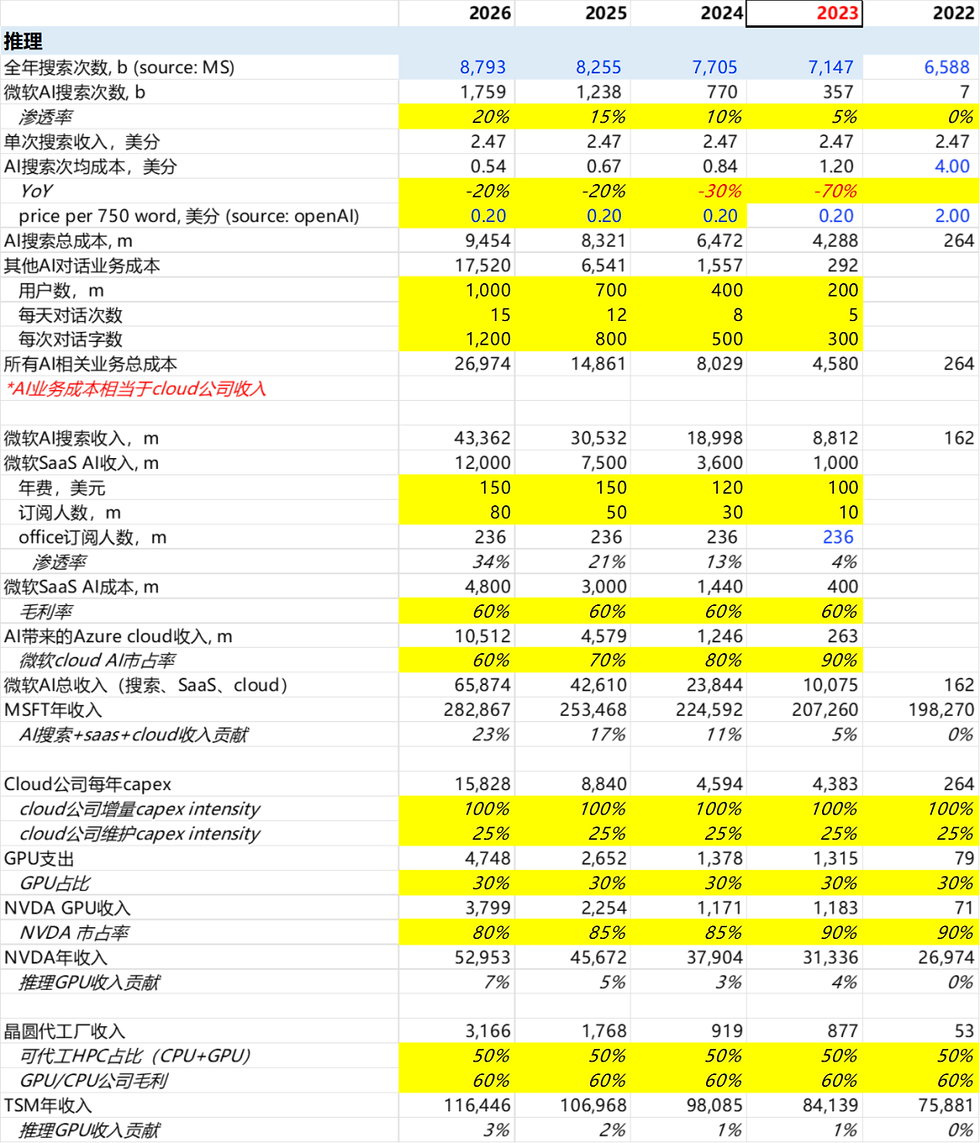
4、appendix 1.NVDA GPU progress speed
Performance comparison between H100 and A100:
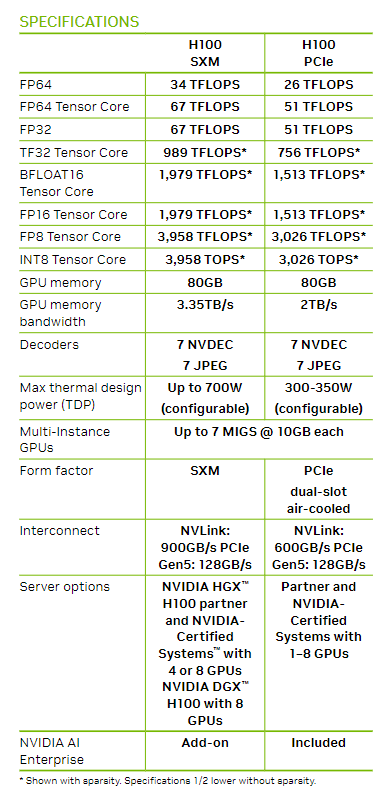
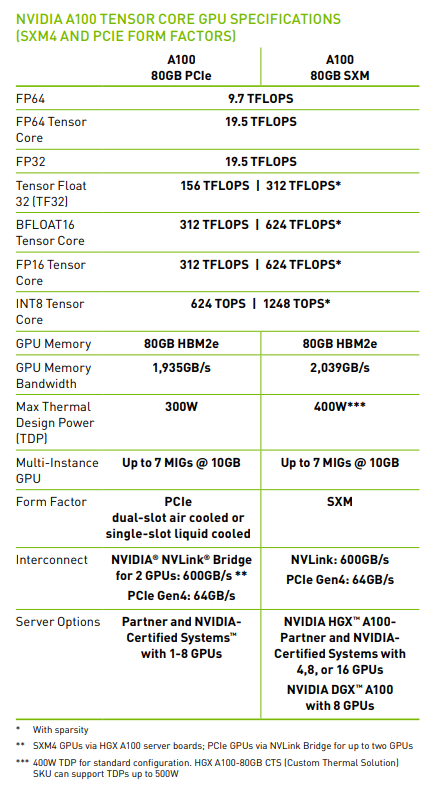
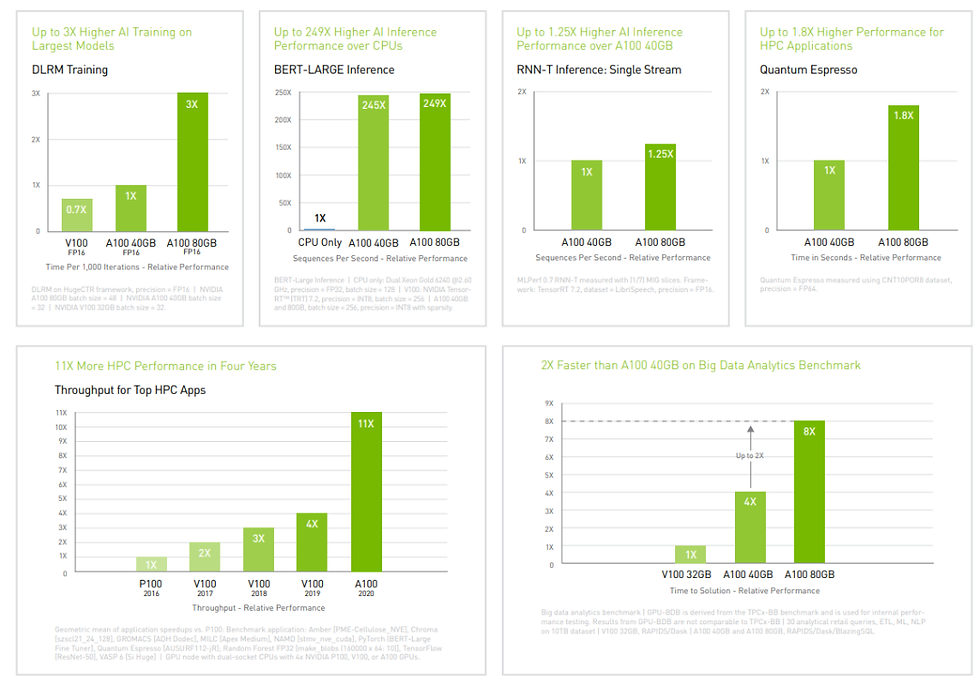
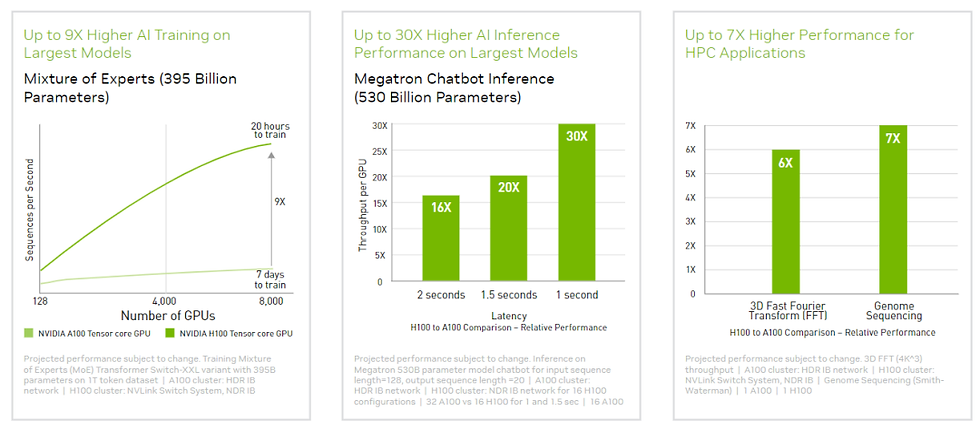
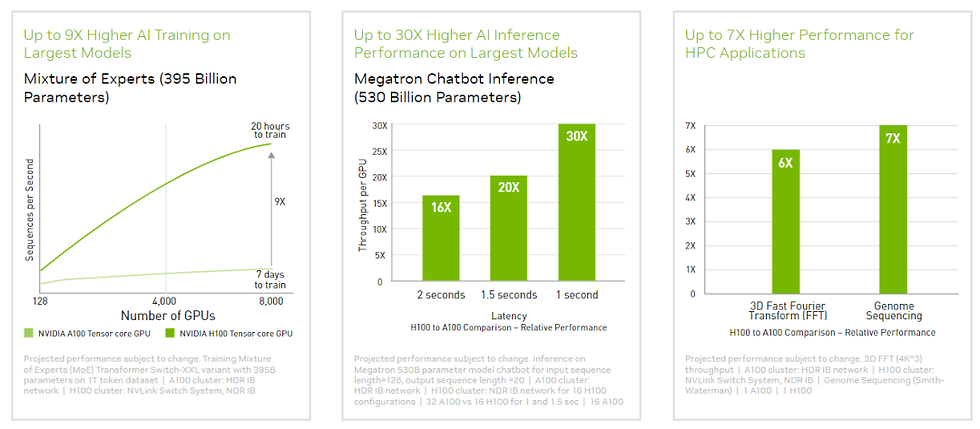
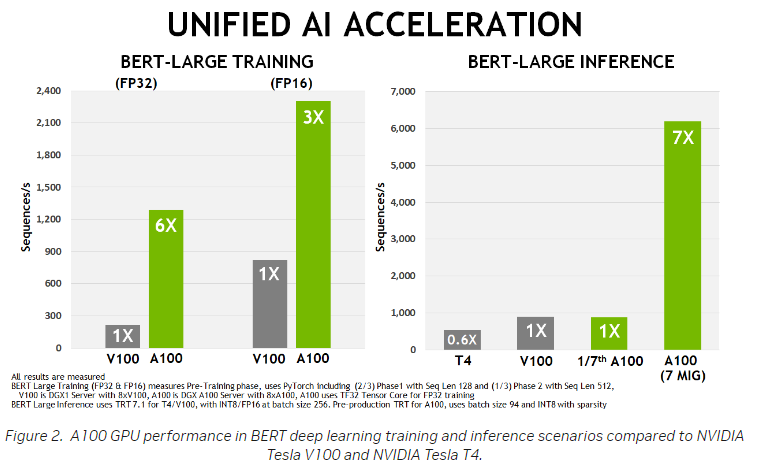
| H100 80G | A100 80G | A100 40G |
发布日期 | 2022 | 2021 | 2020 |
发售价格,美元 | 30,865 | 15,000 | 10,000 |
FP 16,TFLOPS | 1,979 | 624 | 312 |
TFLOPS每美元 | 0.06 | 0.06 | 0.03 |
美元每TFLOPS | 15.60 | 24.04 | 32.05 |
YoY | -35% | -25% | |
Here is the progress of GPU's own performance. Taking into account system-level optimizations (such as NVLink, HBM, etc.), the reduction in computing power cost will be even greater.
Sam Altman claims that a new Moore's Law may soon emerge, in which the amount of intelligence in the universe doubles every 18 months.
2、Technical route
In terms of frameworks: Google's TensorFlow usage is getting lower (4%), while the current mainstream is PyTorch (62%).
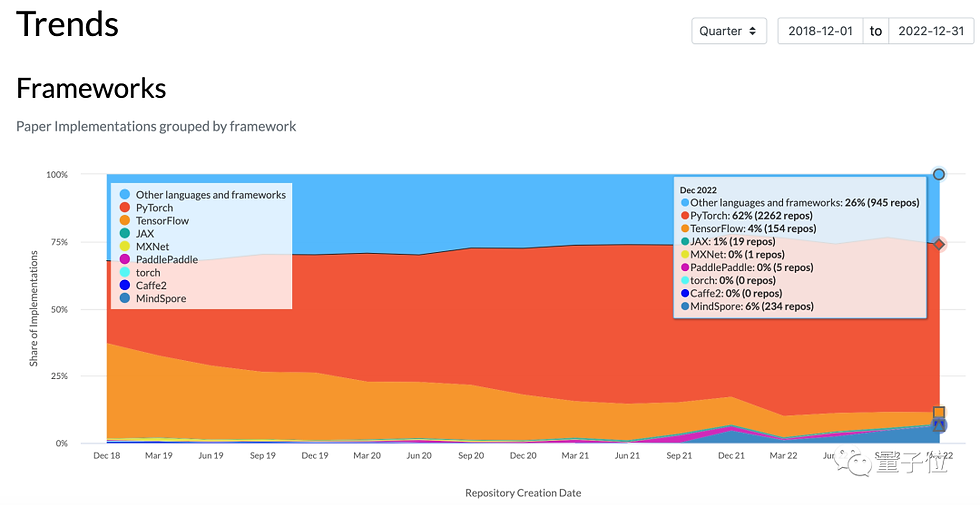
5、Summary:
The number of model parameters for GPT-4 will not increase by hundreds of times as before, but the amount of training data may increase significantly. Coupled with algorithm optimizations, the overall demand for computing power is expected to increase by about 10 times;
The capabilities of large models are expected to continue improving, and new abilities may emerge, leading to a wider range of application scenarios. However, this issue cannot be studied in advance;
AI has the greatest impact on downstream industries. By 2026, the incremental contribution to Microsoft's revenue could reach 20+%.
NVIDIA: The impact in the next two years is not significant. By 2026, the training phase will mainly contribute 2-3% of revenue, and the inference phase will contribute an additional 7% of revenue.
TSMC: The short-term impact is not significant. By 2026, it will only contribute an additional ~4% of revenue. The main influence will be on the demand and rhythm of HPC (High Performance Computing).
Disclaimer: The predictions mentioned above are only the team's conjectures and may have significant forecasting deviations. They should not be considered as investment recommendations or advice.
Comments